We conclude the blog mini series with an article on data challenges that can surface in Citizen Science projects. Citizen Science creates specific risks both in terms of validity of data collected and the ethical issues around data protection. These can be addressed through properly drafted dynamic informed consent forms and validation measures that involve peers, experts and automated systems, or some combination thereof.
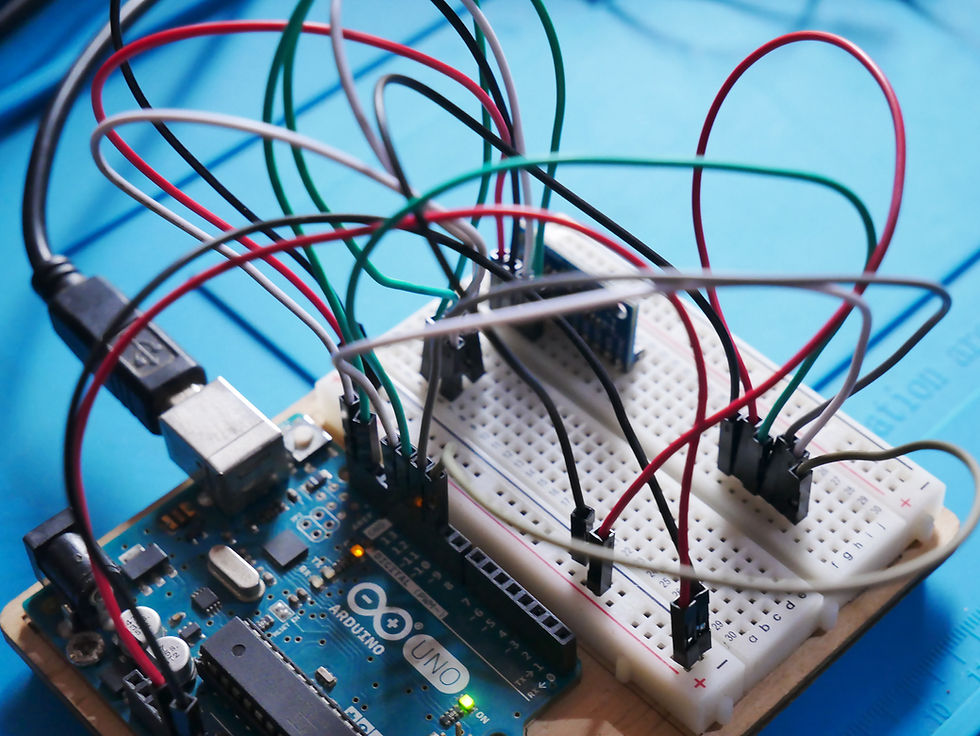
Image courtesy: Unsplash
In air quality monitoring and Citizen Science in general, it’s important to recognize particular motivation aspects when involving the public in data collection. As Hacklay (2018) said, "Citizen Science can open up situations in which participants efforts are exploited or in which projects are conceived without allowing participants to develop deeper engagement even if they wish to do so".
This means, there should be sufficient opening for citizen scientists participating in the experiments to do much more than passive data collection. An example of “passive data collection” could be installing an automated sensor for data collection, requiring little more participation from the citizen scientist than the setup of the sensor to collect data. A loose framework allowing experimentation and participation across different stages will enhance and facilitate deeper engagement during the activity, talking directly into intrinsic motivations of participants. Conversely, limiting the role of participants to mere passive data collectors, can increase the drop-out rate and non-participation.
In this sense, a common guideline is to engage citizen scientists early in the process and continue to engage, including in (sensor) data analysis in an open engagement framework. In COMPAIR, for example, this process started with scoping workshops and will be continued throughout the project. Taking the hypothetical example of the Kiezblock-Network, which could be a part of the COMPAIR case in Berlin using Telraam devices to monitor traffic-impact. There is an opportunity to not only involve citizens in the data collection (installation of sensors) but also interpreting the data post-intervention. This means that citizen engagement is to be extended over a long period of time and participants need to be made aware of the next steps when onboarded.
Vested bias and other issues of ethics
Citizen science creates specific risks both in terms of validity of data collected and the ethical issues around data protection and intellectual property rights.
On the first part, there’s a risk of participation bias which could result in a sample bias of the data collected if there is a correlation between the socio-economic properties of the citizen scientists and the specificity of the data collected. For example, as mentioned earlier, participation in citizen science projects is not equally distributed among the social strata of the population. In the case of air quality, there could be a participation bias with people more conscious about poor air quality, although they are not per se adversely affected and the most adversely affected population groups are typically living in deprived areas (Barnes, 2018), who in turn are typically less engaged in citizen science activities. This issue should be dealt with in the data analysis as sample bias is not unique to citizen science activities and data analysis techniques exist to correct for sample bias. In the spirit of the citizen science activity, this phenomenon creates an opportunity to build awareness on the concept of sample bias and increase knowledge of how to alleviate adverse effects using statistical techniques, together with participating citizens.
Secondly, and more challenging, is a situation when participants knowingly or accidentally tamper with data. For example, an activist participant is participating in a citizen science project with a speed sensor to demonstrate a specific issue of concern (i.e. speeding of passing traffic) and is willfully enabling/disabling sensors collecting this sensor at times the speeding issue is evident (or not). This clearly leads to a bias in the generated data set, moreover in a way that is hard to detect. The latter is an extreme example, but also more implicit behaviour can lead to a similar outcome, for example (re-)activating an air quality sensor at times of noticeable poor air quality or air quality warnings which triggered the participant to (re)activate the sensor.
There are several approaches to mitigate these risks:
A formal engagement clause, a “terms of use/participants” when participants are selected to contribute to the citizen science project. A statement to commit to the project as well as to respect findings, creates a moral barrier, likely sufficient in most cases to mitigate at least active tampering
A sensor/project setup, designed to disable tampering e.g. real-time data collection or rigorous deployment/installation protocols leaving no room to maneuver
Active control by the citizen science project team, verifying if the sensor setup is still within specifications
With respect to ethical issues, key elements to pay particular attention to are privacy, data protection and intellectual property rights. In chapter 20 of The Science of Citizen Science: Ethical challenges and dynamic informed consent (Tauginienė et al, 2021), informed consent is referred to as the point of departure for the description of multiple ethical facets in Citizen Science. Participants need to be fully aware about what they sign up to when participating in a citizen science project. At the very least, this should include:
Rights on personal information, in compliance with the GDPR
Intellectual property of the data collected and analysis done
Terms of use of equipment (e.g. ownership or lending of sensors equipment), or service provided (e.g. licence of the data being generated)
Rewards for service: voluntary contribution and extend of reimbursement of own costs made
The chapter goes on to describe the different types of informed consent, particularly focusing on dynamic informed consent as the solution to the challenges described. There is a conceptual difference between a classic informed consent, which is more transactional in nature versus a dynamic informed consent, evolving as the citizen science activity develops. Broadly summarised, what is referred to as “Ethics v1.0” is a project team led “top down” of information sharing and clearly stated predefined goals of the project to which participants can add few or no changes. By contrast, “Ethics v2.0” is more fluid with continued development and redefined (sub-)project goals - for example specific experiments, or joint/participatory data analysis - that require specific ethical considerations and thus consent form participants. Ethics v2.0 is not static and evolves with the project.
To conclude, any citizen science activity must address diversity and inclusion. In (Paleco, 2021), a full chapter is dedicated to good practices encouraging engagement from all members of society, whatever their social status, sociocultural origin, gender, religious affiliation, literacy level, or age. Recommendations that directly apply to COMPAIR and similar projects include:
Offering multiple project entry points as well as multiple ways to participate at different levels of commitment are key to engaging new and diverse participants. This requires acknowledging that people have very different interests and motivations for engaging in citizen science. In the case of COMPAIR, for example, interaction at different points can be pure data collection with a sensor, or data analysis
Framing research problems as local issues can help to engage individual citizens if they feel a sense of place attachment. This should provide opportunities for the scope of COMPAIR as both traffic concerns and air quality issues are hot topics with a direct personal link to participants
The more project leaders or facilitators participate in actions and are present in the communities affected, the better and the wider community engagement is. Engagement for inclusiveness is typically more labour intensive for the project team. Obviously, there is an important cost trade-off to be made
ethnographic fieldwork prior to engagement i.e. choosing pilot sites in deprived areas for example. This is an opportunity for COMPAIR as poor air quality is mostly an issue of deprived neighbourhoods.
Risks of using false or incorrect information
While sensor quality is not specifically an issue of citizen science (all sensors have quality limitations), particular attention is needed when used in a citizen science project, because of the direct involvement of untrained citizen scientists and the risk of misinterpreting sensor data.
EPA Handbook Citizen Science (EPA 2019) in this respect states “With the advent of new technologies for environmental monitoring and tools for sharing information, citizens are more and more engaged in collecting environmental data, and many environmental agencies are using these data. A major challenge, however, is that data users, such as federal, state, tribal and local agencies, are sometimes sceptical about the quality of the data collected by Citizen Science organisations. One of the keys to breaking down this barrier is a Quality Assurance Project Plan (QAPP).”
In Balázs et al. (2021) authors identify several factors that can undermine quality of data collected by citizen scientists. This can happen when
Data collection protocols are not followed by participants
Data collection protocols do not match the goals of the project
Data collection protocols are incorrectly implemented
Data collection protocols are not comprehensive and are used by stakeholders with different data quality expectation levels
Data used are not fit for purpose
Several options are available to mitigate these risks. Peer verification is an option where collaborating citizen scientists verify observations from colleague citizen scientists. Expert verification is when a scientist with subject matter expertise is involved to affirm or correct findings e.g. by affirming a data analysis done by a participating citizen. Automated verification uses various machine learning techniques to help identify anomalous data points collected by citizen scientists. A more advanced form of this technique is model-based verification which requires a predefined set of algorithms that can a) detect potentially faulty data (similar to automatic verification) and b) automatically attribute a meaningful flag and/or correct errors in citizen science generated data. Setting up a model-based verification requires thorough preparation but once in place can operate fully automatically thereafter.
Various combinations are possible. Machine learning can be used to detect anomalies and then proceed to peer or expert verification in the next step.
Conclusions
We started the blog mini series with a question - What is Citizen Science? From literature review we learned that Citizen Science practice is very diverse. Citizen Science now can be found in (almost) all possible academic disciplines. In that sense, and how it deals with uncertainty in research, Citizen Science cuts through traditional scientific practice. This also leads to the observation that there is no overarching definition of Citizen Science other than the general umbrella of “the public participating in science”. A lot of different meanings exist, often at a project level. However, this doesn’t mean that there are no boundaries of what can be considered Citizen Science.
What all Citizen Science projects have in common is the participation of non-professional scientists in scientific research. This participation, or engagement, can take different forms and apply to all steps of the research process, from problem definition to data collection to dissemination. Furthermore, citizens can participate at different levels. Participation doesn’t always mean the same. Haklay's typology of participation clearly describes these different possible levels of participation of citizens in research projects. The typology also clarifies that the level of participation of a specific project doesn’t have to be fixed and can be changed during the course of a project. A Citizen Science project should find the level of participation most suited for the project at hand. In other words, the participation strategy must be taken into consideration from the start. From the beginning, a Citizen Science project must find its identity between the boundaries of Citizen Science and make clear what is understood by citizen participation.
Some examples can also illustrate the diversity of Citizen Science projects and how participation takes form within. Given that the goals and approaches of each of these projects are different, we can take different lessons from each project about how to tackle participation in Citizen Science. We provided an overview of some important lessons learned from Citizen Science projects regarding the design of a citizen science project and the approach to citizen engagement and its relevance for COMPAIR.
In our project we aim for deep citizen involvement beyond strict data collection. Our ambition is to engage a diverse audience of participants to avoid pitfalls of sample bias. Given the nature of the COMPAIR project with a strong focus on using low-cost sensors, we are developing sound protocols for data validation to maximise the uptake of collected data by policy makers and in other communities.
Bibliography
Balázs B. et. al. (2021). Data Quality in Citizen Science. In K. Vohland, A. Land-Zandstra, L. Ceccaroni, R. Lemmens, J. Perelló, M. Ponti, . . . K. Wagenknecht, The Science of Citizen Science (pp. 139-157). Springer.
Barnes, J, et. al. (2018), Increasing injustice from road traffic-related air pollution in the United Kingdom. Transportation Research D73 (pp56-66). Springer.
Haklay, M. (2018). Participatory Citizen Science. In S. Hecker, M. Haklay, A. Bowser, Z. Makuch, J. Vogel, & A. Bonn, Citizen Science: Innovation in Open Science, Society and Policy (pp. 52-62). London: UCL Press.
Tauginienė, L., Hummer, P., Albert, A., Cigarini, A., Vohland, K. (2021). Ethical Challenges and Dynamic Informed Consent. In: , et al. The Science of Citizen Science. Springer, Cham. https://doi.org/10.1007/978-3-030-58278-4_20
Paleco C. et. al. (2021). Inclusiveness and Diversity in Citizen Science. In K. Vohland, A. Land-Zandstra, L. Ceccaroni, R. Lemmens, J. Perelló, M. Ponti, K. Wagenknecht, The Science of Citizen Science (pp. 261-281). Springer.
EPA (2019) Handbook for Citizen Science - Quality Assurance and Documentation. https://www.epa.gov/participatory-science/quality-assurance-handbook-and-toolkit-participatory-science-projects